TrackGBV: Supervised Learning
Using Artificial Intelligence to Understand Gender-Based Violence
Program Area:
Emerging Tech
Partners:
Location:
12 Pacific Island Countries: Fiji, Solomon Islands, Vanuatu, Tonga, Tuvalu, Marshall Islands, Micronesia, Papua New Guinea, Samoa, Kiribati, Nauru, and Palau
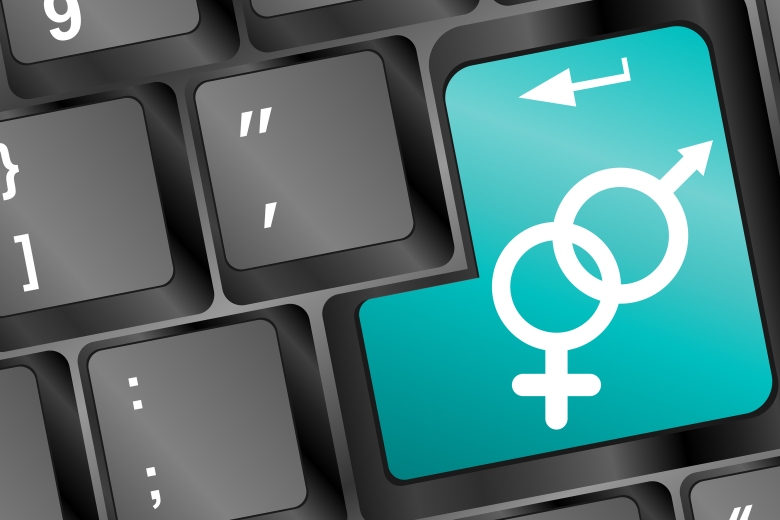
Automation enhances the efficiency of identifying gender-based violence cases and whether judicial decision making is biased.
The Challenge
Evaluating judicial decisions for gender-bias is a necessary endeavor for increasing accountability, but is incredibly time and resource intensive, involving teams of lawyers to identify cases and conduct analysis. ICAAD has been utilizing artificial intelligence to enhance the efficiency of TrackGBV analysis, which has posed a number of challenges.
Experimentation
In trying to identify GBV cases from the corpus of all other legal decisions, various computational approaches were used to evaluate the efficacy of determining relevant GBV cases. The case classification was performed using supervised machine learning. This technique appears to be very efficient in the classification of SA/DV cases (F-score for SA: 96% and DV: 81%) but displayed some limitations in identifying the distinction between relevant and non-relevant cases. Increasing the size of the training set or combining other techniques (e.g. text-mining ) with supervised machine learning algorithms could help refine the classification.
A topic modeling approach was also developed to provide a measure of “emphasis” in identifying key legislation. The limitation of this technique relies on the interpretation of the cosine similarity score, or measure of relative emphasis, and requires close collaboration with legal experts to provide plausible interpretations.
We also used a cluster analysis technique to provide an unbiased overview of the dataset’s trends over time with minimal input. This approach provides a quick assessment of the situation, which was subsequently confirmed with more refined analyses.
Our Solution
Text mining remains an efficient approach to detect occurrences of words within documents and, if providing a good understanding of lexical and grammatical variations for each feature, is an efficient technique for extracting features. However, language evolution requires regular update of the code and a manual verification step is required to estimate the errors made for each of those features.
The conclusions drawn about the effectiveness of different machine learning solutions were obtained solely through the analysis of a case law dataset, which emerges as a valuable source of quantitative information when analyzed with the appropriate tools. The rate of application of legislation, specific usage, trend and distribution of number of cases and offenses, variability in judges’ ruling, and average sentence per charge are examples of data that can be extracted from such dataset and help inform decision makers or advocates on the adherence to legal norms.