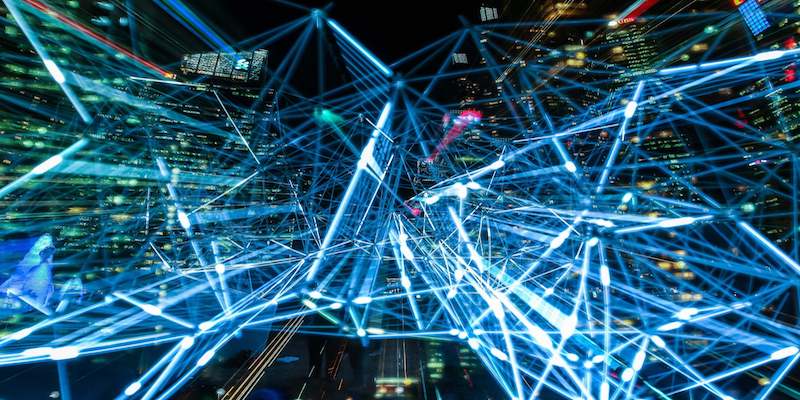
Chris Sexton from our tech firm partner, Conduent, and Greg Tozzi have shared their research from the University of California Berkeley on machine learning techniques to automate ICAAD’s TrackGBV analysis.
They used the 809 Fijian GBV cases from our data set and attempted to classify cases by any language related to gender bias. They used a number of different models, focusing mainly on neural nets, including state of the art approaches BERT, Longformer, and CNN. These were benchmarked against a simpler machine learning approach.
While the precision, recall, and F1 scores hovered in the mid 70% range for all models, the knowledge produced from this research will contribute to ongoing developments. The goal is to develop and apply precise machine learning techniques to monitor judicial bias, increase accountability in the courts, and reduce gender discrimination around the world.
Read the full paper here.