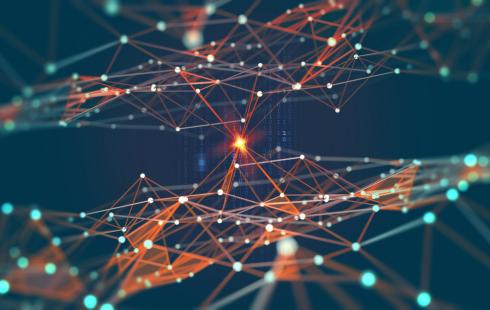
ICAAD recently participated in a workshop by the Partnership on Artificial Intelligence on Explainability in Machine Learning. The idea behind explainable AI is to build accountability, transparency, and trust in the outputs of AI-driven programs. These programs are increasingly applied in our daily lives, from hiring, marketing, and loan approvals, to bail decisions, policing, and housing. Explainable AI is vital to ICAAD’s work, since AI’s application to policy has a profound impact on the rights of the most vulnerable. Explainability is necessary to ensure that people are not unfairly treated, and that if they believe a decision by an AI system was in fact unfair, they can challenge that decision.
Not only is ICAAD engaging with policy efforts to ensure beneficial and explainable AI, we are also reviewing our own internal use of AI. Two of ICAAD’s programs, TrackGBV and TrackSDGs, rely on machine learning techniques to advance our human rights work. We believe it is critical that our datasets are unbiased and that our programs’ use of AI is explainable, especially where governments and civil society organizations are relying on the outcomes of our data-driven approaches.
The recent workshop was convened on Feb. 13th in New York City by the Partnership on AI, an organization established to study best practices in AI, to advance the public’s understanding of AI, and to serve as an open platform for discussion about AI and its influences on society. Representing ICAAD was our AI/Policy Advisor, Jesse Dunietz, PhD. Jesse is a researcher at Elemental Cognition, where he works to define the metrics for the company’s foundational AI research and translate that research into real-world applications. He is also a staff member at the MIT Communication Lab, where he trains STEM graduate students to coach their peers in scientific communication.
At the workshop, participants learned about existing efforts to deploy explainable AI techniques, then discussed how to make such techniques more useful and practical for stakeholders. Jesse’s group examined this question specifically in the domain of social service provision. They considered what various stakeholders might need from explanations of AI decisions, what challenges stand in the way of providing those explanations, and what technical or social advances might help to resolve the challenges.
The Partnership is now working to integrate the discussions from the workshop into its resources and advocacy, aiming to guide both policy and technical research agendas to make explainable AI maximally beneficial to society. In the meantime, ICAAD is already talking with other workshop participants about possible collaborations on AI and human rights projects. What has been underlying ICAAD’s work on AI are the principles of design justice. Going forward, we will be making it clearer how these principles should function in shaping AI policy.